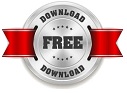
Spoofing attempts in wireless networks and IoT devices can be thwarted if the source of the signal could be identified and blocked. Radiometric identification is also a valuable tool in securing wireless devices. Knowing the source and the brand of the interferer may help identify the offending source. Satellite communication may be faced with intentional or unintentional jamming from rogue sources. The military has been interested in this capability for some time as a means of identifying friendly from hostile radar. Why radiometric identification is of interest are many fold. Such variations, however, are small, imperceptible and difficult to model.
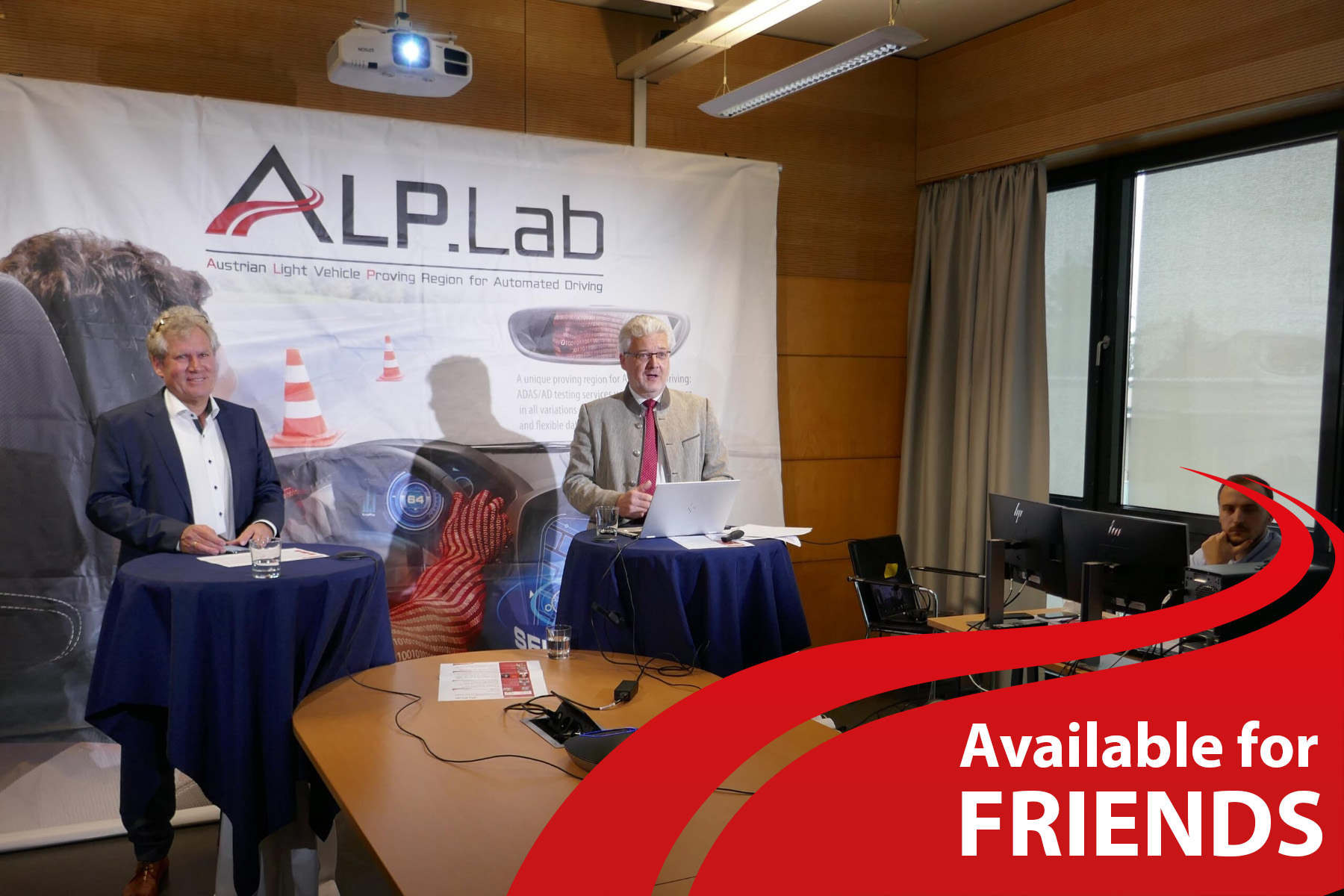
This fact makes subtle device variations the main signature for radiometric identification. In many ways, radiometric identification is a more difficult problem as signals originating from different sources may have similar characteristics such as modulation, bit rates, pulse shapes, etc. However, radiometric identification does not neatly fit in either of the two categories. There is considerable work in signal classification and modulation recognition. Source identification is accomplished by RF fingerprinting of devices by looking for signatures that may arise from manufacturing tolerances, imperfections or normal statistical variations in production. Radiometric identification is the problem of attributing a signal to the source often brand or model. It is shown that the proposed method is simpler to implement, requires no features vectors, needs minimal training and because of its non-iterative structure is faster than existing approaches. The whitening transform is also contrasted with the more recent deep learning approaches that are still dependent on feature vectors with large dimensions and lengthy training phases. Using the Förstner-Moonen measure presents a different perspective compared to maximum likelihood and Euclidean distance metrics. The source is determined by the output of the mode function operated on the Majority Vote Classifier decisions. The whitening transform that produces a data set with the minimum Förstner-Moonen distance to a white noise process is the source signal.
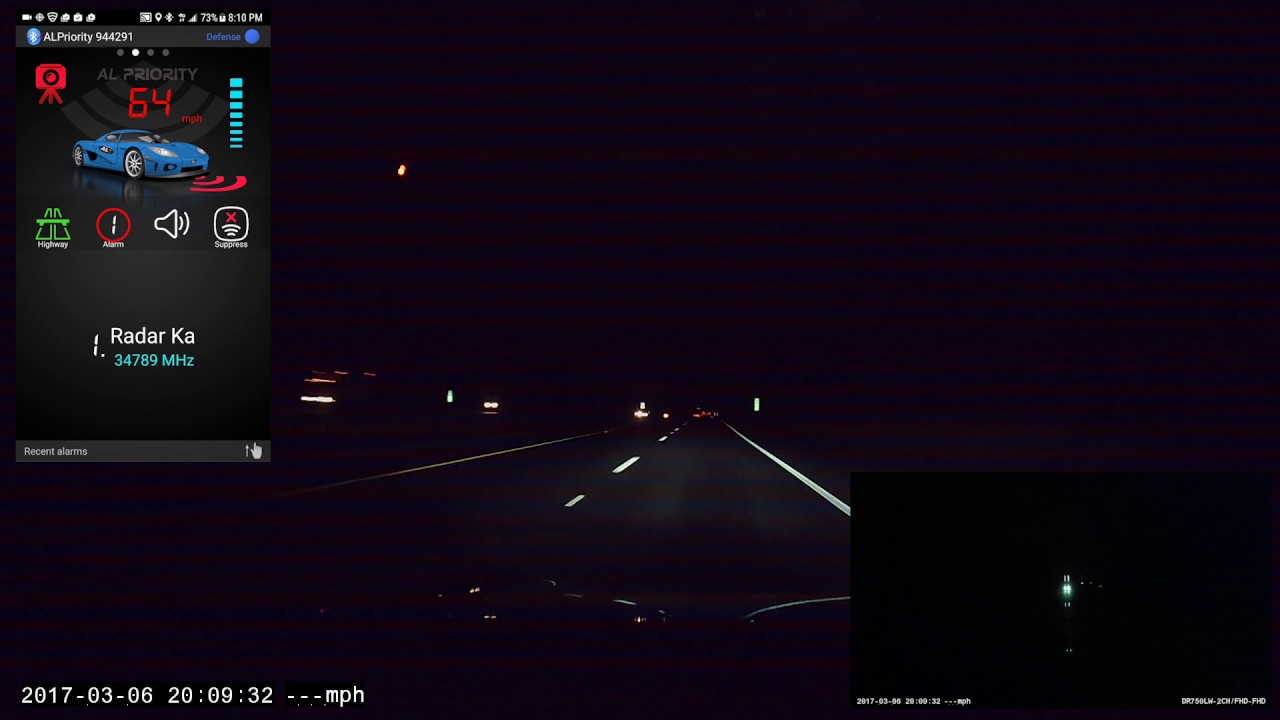
The Förstner-Moonen measure that quantifies the similarity of covariance matrices is used to establish the degree of whiteness. In practice, transformed data are never strictly white since the training and the test data differ. The premise of the idea is that a data set is “most white” when projected on its own whitening matrix than on any other. As such, the commonly used dimensionality reduction algorithms do not apply. The approach stands out from the more established methods in that it works directly on the raw IQ data and hence is featureless. In this work, a radiometric identification algorithm is developed using the whitening transformation. Radiometric identification is the problem of attributing a signal to a specific source.
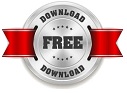